1. AI systems like ChatGPT can produce hallucinations or false information, presenting a significant challenge.
2. Researchers have developed a new method using one AI to detect hallucinations in another AI’s responses.
3. The approach involves asking multiple questions and analyzing the consistency of answers using “semantic entropy.”
4. This method shows improved accuracy in distinguishing correct from incorrect answers compared to previous techniques.
5. While promising, the approach has limitations, including increased energy consumption and inability to address all types of AI hallucinations.
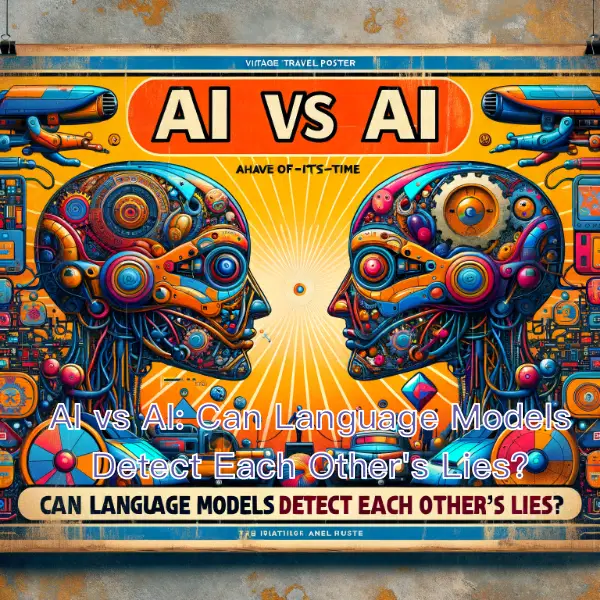
The Rise of AI Lie Detectors: A New Frontier in Machine Learning
In the rapidly evolving landscape of artificial intelligence, a groundbreaking approach has emerged to tackle one of the most persistent challenges in language models: hallucinations. These AI-generated falsehoods have long been a thorn in the side of developers and users alike, but a novel method leveraging the power of AI itself may hold the key to unmasking these digital deceptions.
The Hallucination Dilemma
Artificial intelligence systems, particularly large language models (LLMs) like ChatGPT, have revolutionized how we interact with technology. However, their tendency to produce content that deviates from reality – a phenomenon known as hallucination – has raised significant concerns. From misplacing iconic landmarks to potentially dispensing erroneous medical advice, these AI-generated falsehoods can range from benign to potentially dangerous.
The root of this problem lies in the fundamental architecture of these systems. As AI researcher Andreas Kirsch points out, “There is no difference to a language model between something that is true and something that’s not.” This lack of inherent truth discernment has made the quest to eliminate hallucinations a formidable challenge.
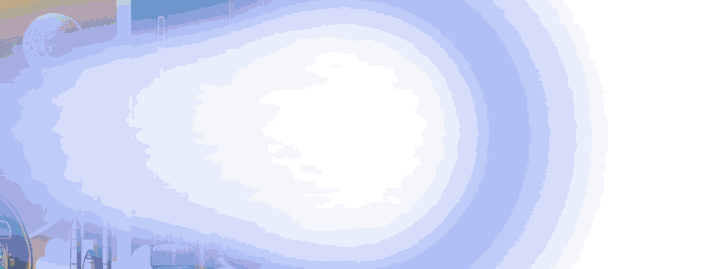
A New Approach: AI Cross-Examination
Enter a team of innovative researchers, including Jannik Kossen from the University of Oxford, who have developed a method that turns AI against itself in the pursuit of truth. Their approach, detailed in a recent Nature study, involves using one language model to interrogate and analyze the outputs of another.
The process works by:
1. Generating multiple responses to the same query from the first AI system.
2. Employing a second AI to group these responses based on semantic similarity.
3. Calculating a measure called “semantic entropy” to assess the consistency and certainty of the grouped answers.
This method effectively mimics the human ability to detect inconsistencies in storytelling, but at a scale and speed only possible with machine learning.
Semantic Entropy: The Key to Unlocking AI Truthfulness
The concept of semantic entropy is central to this new approach. When an AI provides consistent answers across multiple iterations, the semantic entropy is low, indicating a high degree of certainty. Conversely, widely varying responses result in high semantic entropy, suggesting potential confabulation or hallucination.
By leveraging this measure, the system can identify outliers and probable falsehoods with remarkable accuracy. In fact, the study reports a 10% improvement in distinguishing correct from incorrect answers compared to previous methods.
Challenges and Future Directions
While this AI-powered lie detection method shows promise, it’s not without its limitations. The process of generating multiple responses significantly increases energy consumption, raising questions about scalability and environmental impact. Additionally, the approach struggles when an AI lacks the necessary data to answer a question correctly, forcing it to rely on probabilistic guesses.
As Karin Verspoor, dean of the School of Computing Technologies at RMIT University, notes, “We can trust [LLMs] to a certain extent. But there has to be a limit.” This sentiment underscores the ongoing need for human oversight and critical thinking in our interactions with AI systems.
The development of AI systems capable of detecting hallucinations in other AI outputs marks a significant milestone in the field of artificial intelligence. This meta-cognitive approach, where AI essentially fact-checks itself, opens up new avenues for improving the reliability and trustworthiness of language models.
However, this advancement also raises profound questions about the nature of truth and knowledge in the age of AI. As we create increasingly sophisticated systems to validate information, we must grapple with the philosophical implications of machines determining what is “true” or “false.” This could potentially lead to a recursive loop of AI systems checking each other, begging the question: who or what will be the ultimate arbiter of truth?
Moreover, the energy-intensive nature of these validation processes highlights the growing tension between technological advancement and environmental sustainability in AI development. As we push the boundaries of what’s possible with machine learning, we must also innovate in ways that minimize our ecological footprint.
Ultimately, while AI-powered lie detection represents a promising step forward, it also serves as a reminder of the complex challenges that lie ahead in our quest to create truly reliable and ethical artificial intelligence systems.
Free AI Research Guidebook: